Operationalize data and AI platform
Operationalize the data and AI platform and data science model means moving the data science models and analytical application into production. Also, this includes managing the models, overseeing the data platform/data pipes, and maintaining analytical business applications.

Steps for Operationalizing a Data and AI Platform
Operationalizing a data and AI platform involves taking a data and AI solution from development and testing to production and ongoing maintenance. Furthermore, this process ensures that the solution is reliable, scalable, and secure. Additionally, it can be effectively monitored and maintained over time
Data and AI Governance
Firstly, establish clear governance policies and procedures. Moreover, this also includes roles and responsibilities, data management and security processes. Additionally, standards and guidelines for data quality, compliance, and privacy is essential.
Infrastructure and Architecture
Similarly, set up the necessary hardware, software, and cloud resources. Furthermore, design the data pipeline and data storage architecture to support the platform.
Monitoring and Management
Regularly monitor the performance of the platform and data pipeline, data storage, and machine learning models. Additionally, to identify and address any issues in a timely manner.
Maintenance and Upgrades
Additionally, perform regular updates, backups, and disaster recovery procedures. Likewise, to keep the platform running smoothly and up to date with the latest technologies and best practices.
Scalability
Firstly, design the platform to handle high volumes of data. Furthermore, implement automatic scaling and load balancing to adapt to changing demands.
Security
Implement security best practices such as encryption, firewalls, and access controls. Additionally, this is to ensure that data is protected, and that only authorized users can access the platform.
FAQs
Operationalizing a data and AI platform involves taking a data and AI solution from development and testing to production and ongoing maintenance. Additionally, this ensures that the solution is reliable, scalable, and secure, and that it can be effectively monitored and maintained over time.
Data governance is important when operationalizing a data and AI platform because it establishes clear policies and procedures for managing data. Likewise, this also includes roles and responsibilities, data management and security processes, and standards and guidelines for data quality, compliance, and privacy. Additionally, to identify and address any issues in a timely manner.
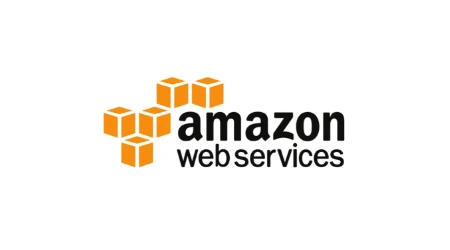
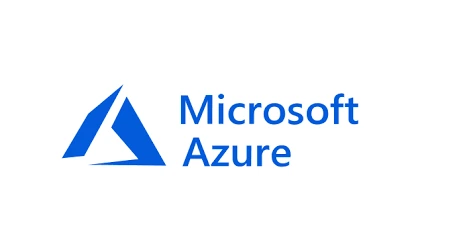

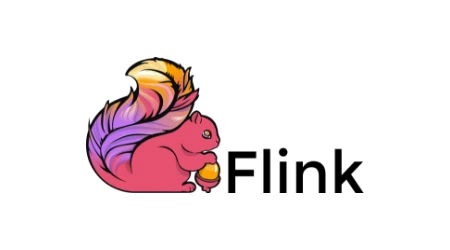

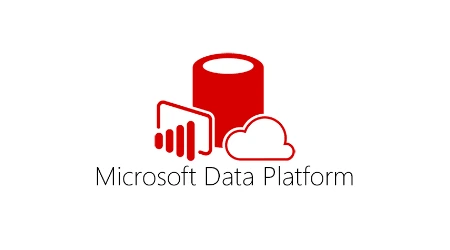

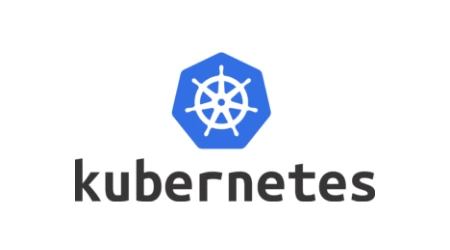

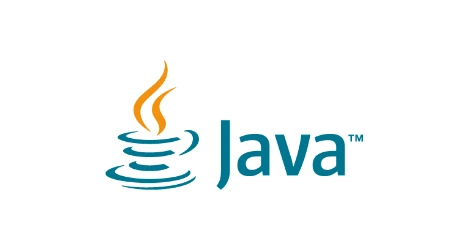
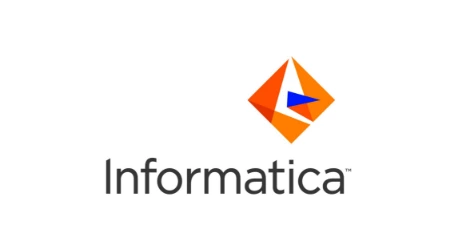
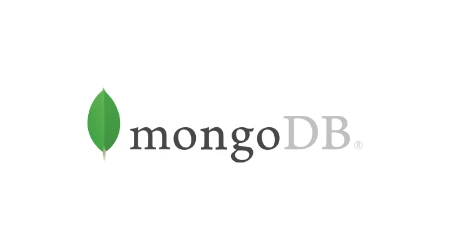

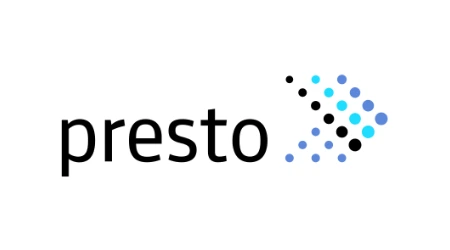

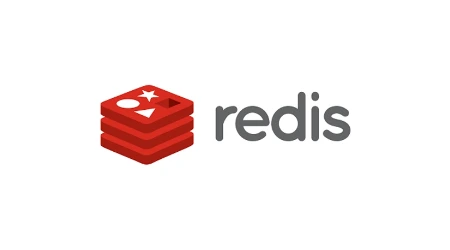


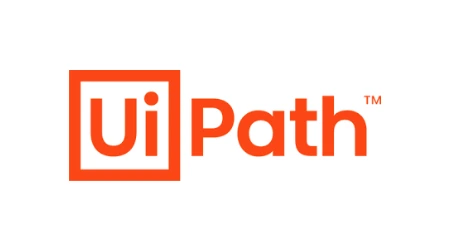

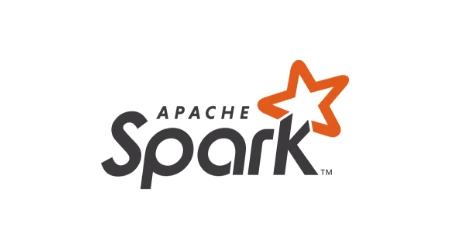
Case Studies
Unlock the Potential of Data Science with Aptus Data Labs
Don't wait to harness the power of data science - contact Aptus Data Labs today and start seeing results.
Are you planning to take your business to the next level with data science? We invite you to connect with us today to schedule a consultation. Our team will work with you, to assess your current data landscape and develop a customized solution that will help you gain valuable insights and drive growth.